Artificial intelligence continues to evolve rapidly, introducing innovations that affect various aspects of life and work. Recent advancements include improved natural language processing, enhanced machine learning models, and breakthroughs in computer vision. These developments open new opportunities for businesses and individuals alike.
You can expect to see AI systems that communicate with greater nuance and understand context more effectively. Applications in healthcare, finance, and creative industries are becoming smarter and more efficient, providing solutions that were once considered science fiction.
Staying informed about the latest trends in AI is essential, as these technologies are reshaping how we interact with the digital world. By understanding what’s new, you can better leverage these advancements to enhance your skills and productivity.
Advancements in Machine Learning Algorithms
Recent developments in machine learning algorithms are shaping the landscape of artificial intelligence. Key areas of focus include deep learning, transfer learning, and reinforcement learning, each contributing significantly to the advancement of Artificial Intelligence capabilities.
Deep Learning Evolution
Deep learning continues to evolve, driven by more sophisticated architectures and increased computational power. Notable frameworks like TensorFlow and PyTorch support complex neural networks, enabling models to learn from vast datasets.
Attention mechanisms, especially in the form of transformers, have revolutionized natural language processing. These mechanisms allow models to weigh the importance of different words, improving contextual understanding in tasks such as translation and summarization.
Furthermore, advancements in hardware, including GPUs and TPUs, facilitate faster training times, allowing for more extensive experimentation and refinement of deep learning models.
Transfer Learning Progressions
Transfer learning has gained traction as a method for leveraging pre-trained models to tackle new tasks with limited data. This approach significantly reduces training time and resource allocation.
Fine-tuning pre-trained models allows you to adapt established architectures for specific applications, enhancing performance while minimizing data requirements. Techniques such as domain adaptation make it easier to apply models across different contexts.
Popular pre-trained models, like BERT and GPT, illustrate the effectiveness of transfer learning. You can utilize these models for various applications, from text classification to even image recognition tasks.
Reinforcement Learning Breakthroughs
Reinforcement learning (RL) has seen breakthroughs that enhance decision-making capabilities in dynamic environments. Algorithms such as Proximal Policy Optimization (PPO) and Deep Q-Networks (DQN) optimize strategies by learning through trial and error.
Recent advancements include the application of RL in complex areas like robotics and game playing. These models can adapt to changes in their environment, improving their efficiency and effectiveness.
Additionally, integration with neural networks has led to more sophisticated RL applications. This combination enhances the ability of AI systems to navigate challenging tasks, making them more versatile across industries.
Natural Language Processing Innovations
Recent developments in natural language processing (NLP) are reshaping how machines understand and interact with human language. Key innovations include transformative language models, advancements in sentiment analysis, and progress in semantic search.
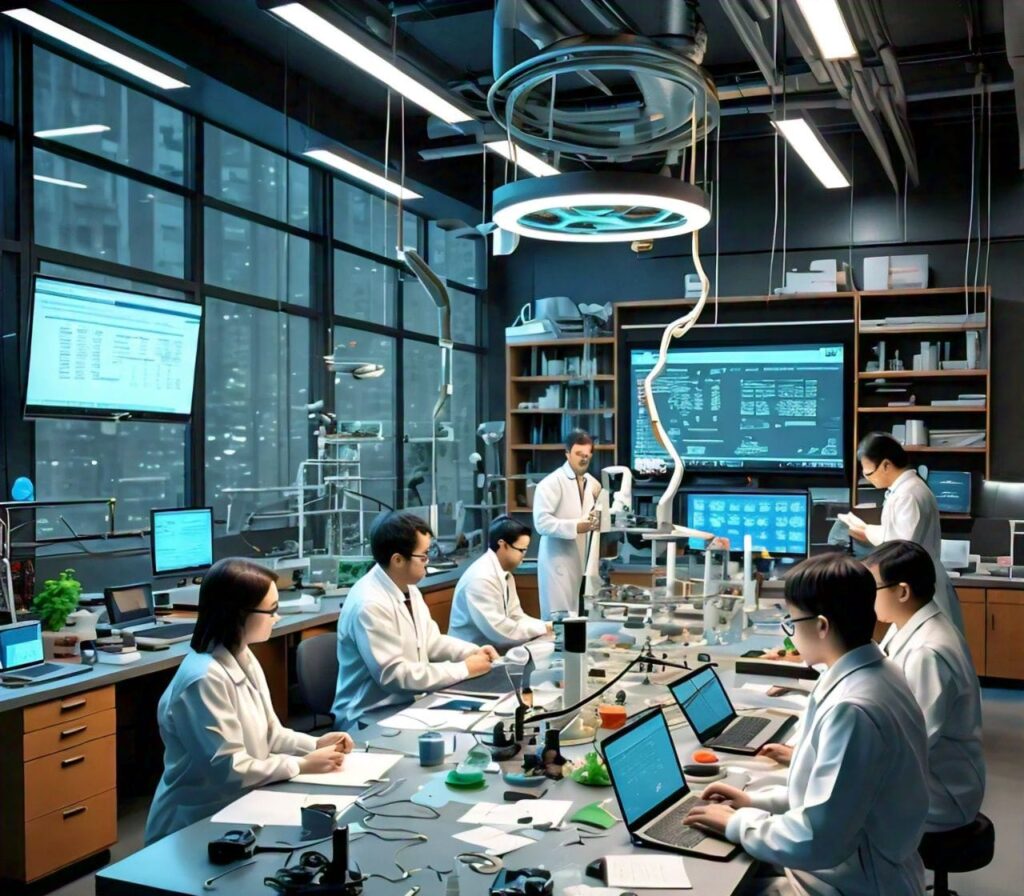
Transformative Language Models
The rise of large language models like GPT-4 has significantly impacted NLP capabilities. These models utilize billions of parameters to generate text that closely mimics human writing. By training on diverse datasets, they understand context, grammar, and even nuances in tone.
Key Features:
- Context Awareness: Improved retention of context over longer texts.
- Generative Capabilities: Ability to create coherent and contextually relevant content.
- Task Versatility: Effectively performs various tasks, from summarization to translation.
Businesses leverage these models for chatbots, content creation, and automated customer support, improving efficiency and user experience.
Advances in Sentiment Analysis
Sentiment analysis has advanced with enhanced algorithms that decode emotions embedded in text more accurately. Modern tools identify not just positive or negative sentiments but also capture underlying feelings such as joy, anger, or frustration.
Main Components:
- Aspect-Based Analysis: Allows for a granular approach to understanding sentiments related to specific features or aspects.
- Multimodal Inputs: Incorporates data from social media, reviews, and other platforms for comprehensive insights.
- Domain Adaptability: Tailored models can switch between industries, offering insights relevant to specific markets.
These advancements support businesses in monitoring brand reputation and customer engagement, enabling data-driven strategies.
Progress in Semantic Search
Semantic search represents a shift from keyword-based queries to context-driven searches. This innovation focuses on understanding user intent and the meanings behind search terms.
Benefits Offered:
- Improved Relevance: Users receive results that are contextually relevant rather than just a match for keywords.
- Natural Language Queries: Supports conversational search, allowing users to ask questions as they would in dialogue.
- Integration of Knowledge Graphs: Links entities and concepts for richer, more informative responses.
Enhancements in semantic search help organizations provide more accurate information, improving user satisfaction and engagement.
1. Generative AI Advancements: Shaping the Future of Creativity
Generative AI has emerged as one of the most transformative technologies in recent years, fundamentally changing how we think about creativity, content creation, and communication. This branch of artificial intelligence is designed to produce new content—text, images, music, or video—by learning patterns from existing data. As we dive deeper into the advancements in generative AI, it becomes clear that its implications are far-reaching, influencing various sectors and redefining traditional creative processes.
Understanding Generative AI
At its core, generative AI utilizes complex algorithms, particularly those based on deep learning, to generate new content. These models are trained on large datasets, allowing them to learn the nuances of language, imagery, or sound. For example, a generative text model like GPT-4 can produce coherent and contextually relevant essays, articles, or even poetry by predicting the next word in a sequence based on prior context. Similarly, image generation models like DALL-E can create stunning visuals from textual descriptions, offering limitless creative possibilities.
Recent Breakthroughs
Enhanced Creativity and Customization
One of the most notable advancements in generative AI is its ability to personalize content. Recent developments have enabled these systems to create tailored outputs based on user preferences. For instance, marketing teams can utilize generative AI to develop bespoke advertisements that resonate more deeply with target audiences. By analyzing data on consumer behavior and preferences, AI can produce unique content that not only captures attention but also drives engagement.
Additionally, tools like Canva have integrated AI-powered features that allow users to generate design elements based on simple prompts, making graphic design accessible to those without technical skills. This democratization of creativity empowers individuals and small businesses to produce professional-grade content quickly and efficiently.
Integration with Other Technologies
The convergence of generative AI with other technologies is also a significant trend. For instance, AI-generated content is increasingly being combined with augmented reality (AR) and virtual reality (VR) to create immersive experiences. Imagine a virtual environment where users can interact with AI-generated characters that respond in real time, offering personalized storytelling or educational experiences. This integration opens new avenues for entertainment, education, and even therapy, creating dynamic interactions that were previously unimaginable.
Improvements in Quality and Realism
Another breakthrough is the enhanced quality and realism of generated content. Earlier models often produced results that lacked coherence or realism. However, advancements in neural networks, particularly those leveraging transformer architectures, have dramatically improved output quality. These models can now generate text that closely resembles human writing and images that are visually stunning and lifelike.
For instance, AI can create artwork that mimics the style of famous painters or generate photorealistic images based on abstract concepts. This capability not only showcases the potential of generative AI in creative fields but also raises questions about authorship and originality, challenging our understanding of what it means to be creative.
2. AI in Healthcare: Transforming Patient Care
Artificial intelligence (AI) is making significant waves in the healthcare industry, reshaping how medical professionals diagnose, treat, and manage patient care. With its ability to analyze vast amounts of data quickly and accurately, AI is enhancing the way healthcare providers work, leading to better outcomes for patients. In this post, we will explore how AI is being used in healthcare, highlighting its benefits and potential challenges.
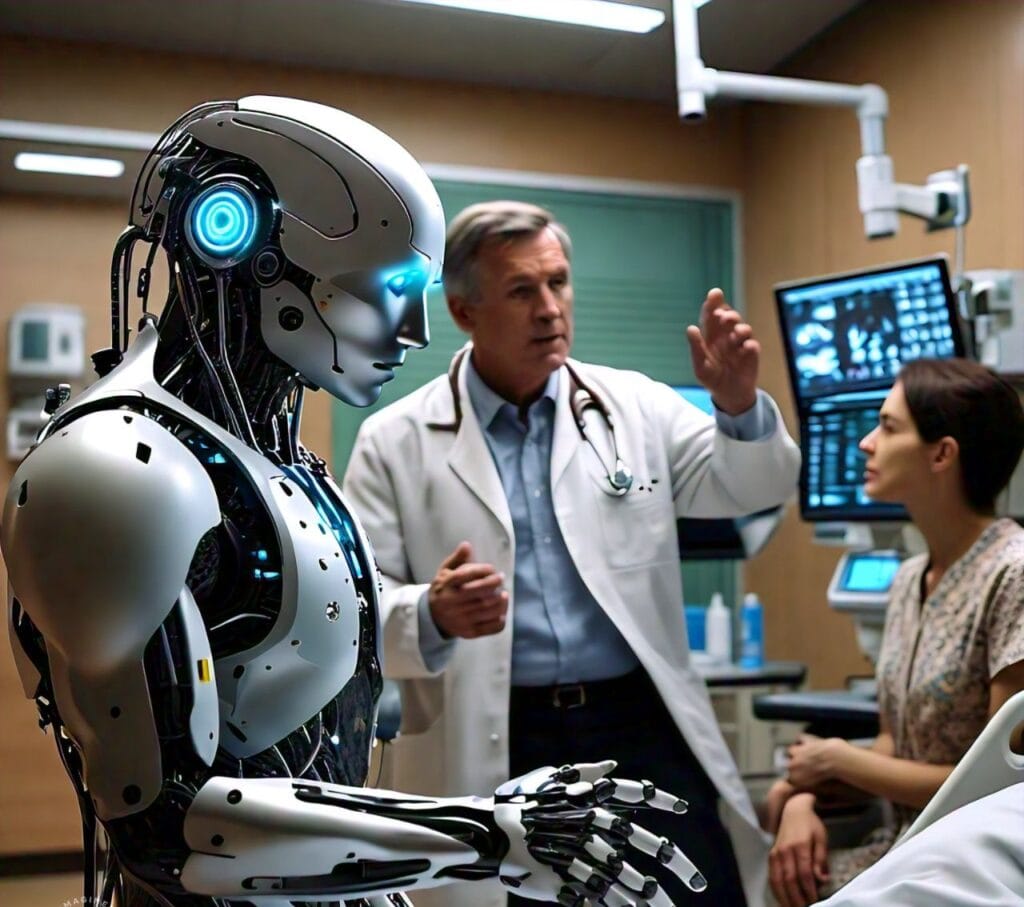
How AI Works in Healthcare
At its core, AI refers to computer systems that can perform tasks that typically require human intelligence. This includes learning from data, recognizing patterns, and making decisions. In healthcare, AI can analyze medical records, lab results, imaging scans, and even genetic information. By processing this data, AI systems can assist doctors in making more informed decisions about patient care.
Diagnostic Support
One of the most exciting applications of AI in healthcare is its role in diagnostics. AI algorithms can analyze medical images, such as X-rays, CT scans, and MRIs, to identify abnormalities like tumors or fractures. For example, studies have shown that AI systems can detect certain types of cancers as accurately as trained radiologists. This capability can lead to earlier diagnoses, which are crucial for effective treatment.
Additionally, AI can assist in diagnosing diseases by analyzing symptoms and patient history. Chatbots and virtual health assistants can gather patient information through simple conversations, helping healthcare providers understand a patient’s condition better before an in-person visit.
Personalized Treatment Plans
AI is also paving the way for personalized medicine. By examining a patient’s genetic information and health records, AI can help doctors create tailored treatment plans. This means that instead of a one-size-fits-all approach, treatments can be customized to fit an individual’s specific needs, leading to better results.
For instance, in cancer treatment, AI can analyze data from various sources, including previous treatments and outcomes, to suggest the most effective therapies for a particular patient. This personalized approach can reduce side effects and improve the overall effectiveness of treatments.
Predictive Analytics
Another important application of AI in healthcare is predictive analytics. By examining historical data, AI can identify trends and predict future health outcomes. For example, hospitals can use AI to forecast patient admissions, allowing them to allocate resources more effectively and reduce wait times.
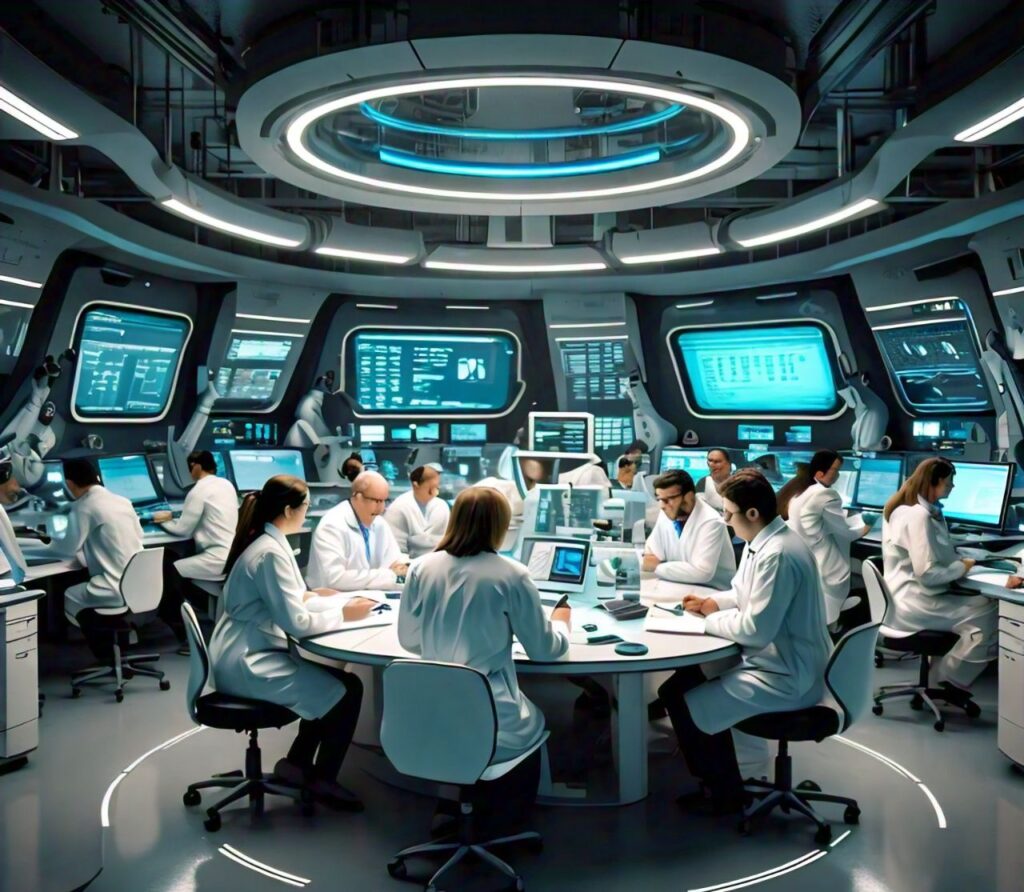
In chronic disease management, AI can analyze data from wearable devices and health apps to predict potential health crises. This proactive approach enables healthcare providers to intervene early, helping to prevent emergencies and improve patient outcomes.
3. Ethical AI and Regulation: Ensuring Responsible Use
As artificial intelligence (AI) becomes a bigger part of our lives, the need for ethical guidelines and regulations is more important than ever. AI can bring many benefits, but it also raises serious questions about fairness, privacy, and accountability. In this article, we’ll explore what ethical AI means, why it’s crucial, and how regulations can help ensure that AI is used responsibly.
Understanding Ethical AI
Ethical AI refers to the principles and guidelines that govern how AI systems should be developed and used. The main goal is to make sure that AI technologies are fair, transparent, and accountable. This means that AI should treat all people equally, be easy to understand, and have clear consequences for its actions.
For example, when AI is used in hiring processes, it should not discriminate against candidates based on their gender, race, or age. Instead, it should focus on a person’s skills and qualifications. By adhering to ethical principles, developers can build AI systems that enhance society rather than harm it.
The Importance of Ethical AI
- Fairness: One of the biggest concerns with AI is bias. If AI systems are trained on biased data, they can make unfair decisions. For instance, an AI used for loan approvals might unfairly deny applications from certain groups of people. Ensuring fairness means actively working to eliminate biases in AI algorithms and datasets.
- Transparency: AI can often be a “black box,” meaning that it’s difficult to understand how it makes decisions. Transparency is crucial so that users and stakeholders can understand why an AI system reached a specific conclusion. This helps build trust and allows people to hold AI accountable.
- Privacy: AI systems often rely on large amounts of personal data. Protecting this data is vital to ensure people’s privacy. Ethical AI practices involve implementing strong data protection measures and only using data with the consent of individuals.
- Accountability: When AI makes mistakes or causes harm, it’s important to know who is responsible. Clear accountability helps ensure that developers and companies take responsibility for their AI systems and their impacts.
The Role of Regulation
To promote ethical AI, governments and organizations are beginning to introduce regulations. These rules are designed to ensure that Artificial Intelligence is developed and used in ways that align with ethical principles. Here are some key areas where regulation is making an impact:
- Standards and Guidelines: Regulatory bodies are creating standards for AI development. These guidelines help developers understand the best practices for building ethical AI systems. For example, they may outline how to conduct bias assessments or ensure transparency in algorithms.
- Data Protection Laws: Many countries have implemented laws to protect personal data, such as the General Data Protection Regulation (GDPR) in Europe. These laws require companies to handle data responsibly, ensuring that individuals have control over their personal information.
- Risk Assessments: Some regulations require companies to conduct risk assessments before deploying AI systems. This means analyzing potential harms and taking steps to mitigate them. For example, before launching a facial recognition system, a company may need to evaluate how it could impact privacy and civil rights.
- Public Consultation: Engaging the public in discussions about AI policies is becoming more common. By seeking input from diverse groups, regulators can better understand the concerns and needs of different communities, leading to more effective regulations.
Which Technology Is Used in iPhones: 5 Revolutionary Technologies Behind the iPhones You want to learn more about this.
Conclusion
The world of artificial intelligence is rapidly evolving, bringing exciting breakthroughs and trends that are changing how we live and work. From generative AI’s ability to create unique content to its growing role in healthcare for better diagnoses and personalized treatments, these advancements hold great promise. Additionally, the focus on ethical AI and regulation ensures that as we innovate, we also prioritize fairness and accountability. Staying informed about these developments helps us understand the potential of AI and how it can positively impact our lives in the future.